Using neural networks to predict traffic flow
Yutian Liu defended her PhD thesis at the Department of Built Environment on May 13th.
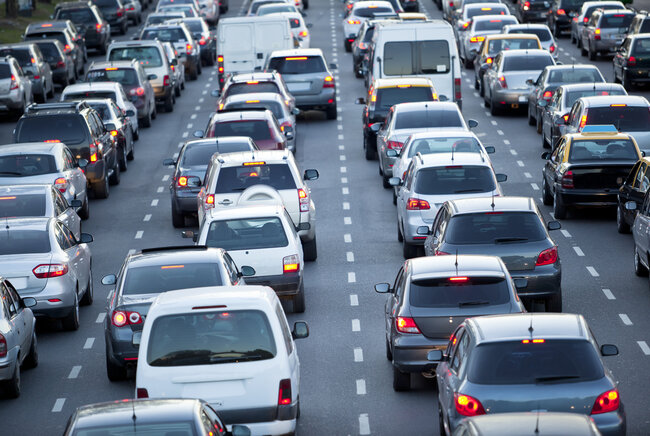
People rely on robust and accurate traffic information to make decisions for route choice and traffic management. Traffic prediction, which relies on historical data to predict future traffic conditions, faces significant challenges due to the dynamic and intricate spatiotemporal features. The advancement of big data and computational power have driven graph based deep learning models to the forefront of traffic prediction solutions. For her PhD research, Yutian Liu focused on developing innovative solutions to overcome these challenges and improve the accuracy and reliability of traffic predictions.
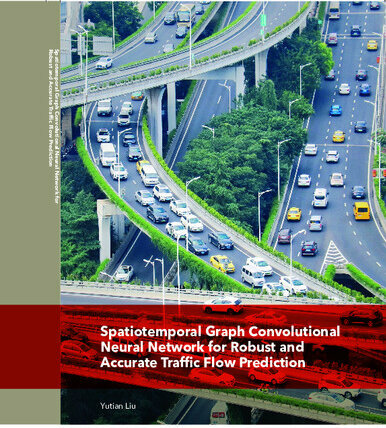
However, existing studies related to GCN-based (Graph Convolutional Network) traffic flow prediction models have limitations, including the static assumption of network structures and reliance on precise historical data. These limitations neglect model robustness under imperfect datasets.
Proposed solutions
To tackle these challenges, Yutian Liu’s research first proposes the ST-DAGCN (Spatiotemporal Dual Adaptive Graph Convolutional Network) model. This model utilizes a dual-adaptive adjacency matrix to learn global static and local dynamic traffic features.
Enhancing robustness
Secondly, to enhance the model’s robustness in handling noisy/missing data, Liu introduces the RT-GCN (Robust Spatiotemporal Graph Convolutional Network). This novel traffic prediction model is designed to manage noisy and missing data by employing a Gaussian distributed node representation along with a variance-based attention mechanism.
Strengthening existing models
Lastly, in this research, Liu designs a method called Sparse AT (Sparse Adversarial Training) to strengthen the robustness of existing deep learning models used in traffic forecasting. It utilizes a min-max optimization algorithm to enhance the models’ robustness.
In conclusion, this thesis contributes to the advancement of deep learning models for traffic flow prediction.
Title of PhD thesis: Spatiotemporal Graph Convolutional Neural Network for Robust and Accurate Traffic Flow Prediction. Supervisors: Soora Rasouli, Tao Feng, and Melvin Wong.