Optimizing Deep Learning for MRI segmentation
Yasmina Al Khalil defended her thesis at the Department of Biomedical Engineering on November 16.

Magnetic resonance imaging (MRI) plays a crucial role in diagnostics, obtaining detailed images of the human body. The growing need for efficient data analysis has led to the emergence of automated techniques, particularly deep learning, for MRI image segmentation.
With her PhD research, Yasmina Al Khalil focused on reducing acquisition dependence in MRI image segmentation, focusing on the development and optimization of deep learning algorithms. She focuses on addressing the challenges of variability in MRI acquisition protocols and uses both physical and deep learning-based data generation methods to improve robustness and generalization of trained segmentation algorithms. The research also explores data expansion, pre- and post-processing techniques and changes in network architecture to improve adaptation under limited data availability.
Deep learning algorithms
Key findings from Al Khalil's research highlight the impact of MRI acquisition variability on segmentation performance and present strategies to reduce this dependency. By focusing on data-driven development, it offers insights to create robust algorithms that are less dependent on specific sequences, for practical implementation in clinical settings.
With her research, Al Khalil provides a critical evaluation of deep learning algorithms for MRI image segmentation, with valuable insights for further development of automated analysis methods in medicine, focusing on a data-driven approach and reducing the complexity of generalization in medical image segmentation.
Title of PhD thesis: “Reducing acquisition dependency in magnetic resonance image in magnetic resonance image”
Supervisors: Marcel Breeuwer and Josien Pluim
Latest news
- News Overview
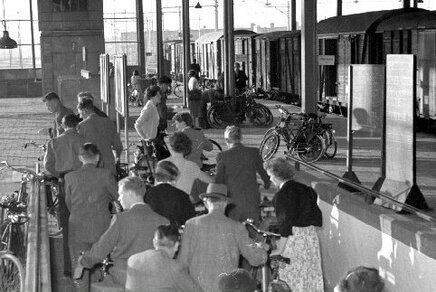
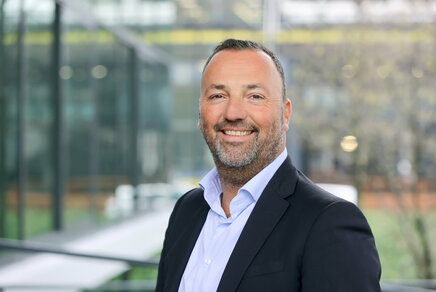