Generating large amounts of artificial images for data-hungry deep learning algorithms
Sina Amirrajab defended his thesis at the Department of Biomedical Engineering on April 20th.
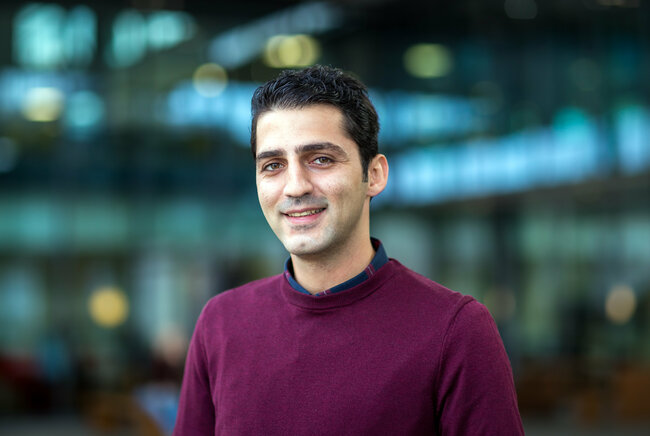
Cardiovascular disease, which refers to the range of diseases affecting the heart and blood vessels, is the leading cause of death worldwide. Magnetic resonance imaging has become an essential tool in cardiology, allowing clinicians to noninvasively assess the anatomy and function of the heart. In his thesis, Sina Amirrajab has developed several frameworks and pipelines for simulation and synthesis of cardiac magnetic resonance images. His goal is to generate large numbers of images with corresponding labels that can be used to develop automated image segmentation methods based on deep neural networks.
To calculate volumes and flow rates at different stages of the cardiac cycle, a first critical step is segmentation: accurate delineation of cardiac structures. Although fully automated cardiac image segmentation methods exist, they are not yet accurate enough for clinical use. Therefore, semi-automated approaches are currently preferred. Recent advances in deep learning (DL), a subfield of artificial intelligence, hold promise for improving automated image segmentation in cardiac image analysis.
However, a major challenge is the limited availability of high-quality medical data with ground truth labels. This makes it difficult to develop data-hungry deep learning algorithms for automated image segmentation. Collecting, annotating and sharing medical data with the desired quality and variation is not always feasible, but it is essential for the development of such algorithms. To facilitate the development of such methods, the main theme of this research was to investigate and propose different solutions for generating large amounts of artificial images with ground truth labels.
In particular, Amirrajab presents solutions for two main categories of image generation, namely physics-driven image simulation and data-driven image synthesis. The former relies on the underlying physics of magnetic resonance imaging based on the so-called Bloch equations to simulate data, while the latter applies recent algorithms known as generative models to learn imaging features and anatomical characteristics from abundant data and in turn synthesize new images.
In summary, several studies presented in his thesis demonstrate the benefits of simulating and synthesizing cardiac magnetic resonance images with corresponding data augmentation labels to improve performance and address medical data scarcity in the context of developing deep-learning supervised medical image analysis algorithms. Amirrajab believes that his research will play a critical role in the development of image simulation and synthesis platforms for studies on cohorts of artificially generated virtual subjects. It is also important for the development, optimization and benchmarking of a number of medical image analysis algorithms, including but not limited to deep neural networks for automated image segmentation.
Title of PhD thesis: “Simulation and Synthesis for Cardiac Magnetic Resonance Image Analysis”
Supervisors: Marcel Breeuwer and Josien Pluim