Adaptable AI systems to keep pace with the evolving world
New methods developed by Ghada Sokar impact every facet of our lives.
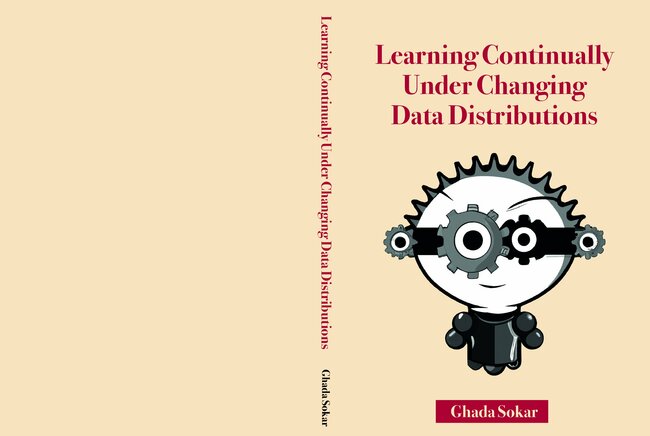
Artificial Intelligence (AI) has seamlessly become an integral part of our daily routines, with influence across diverse domains such as healthcare, robotics, and education, contributing to advancements and innovations. However, the current AI systems face a critical limitation: they struggle to adapt to the rapid and continuous changes in data. This adaptability is the key to pushing AI to the next level, facilitating meaningful and positive transformations in our lives. PhD student Ghada Sokar has developed new methods that empower AI systems with the ability to learn continuously, adapting to new and evolving data. She defended her thesis on October 31.
Adaptability is crucial for AI systems to keep pace with the evolving world around us. Climate change, for instance, demands accurate weather predictions, necessitating AI models that swiftly adapt to new climate patterns and data. The constant influx of images and videos on social media alters our preferences, calling for recommendation systems that can promptly adjust to our shifting behaviors, ensuring a tailored and efficient user experience.
Similarly, in healthcare, the ability to adapt to new diseases, symptoms, and treatments is vital for enhancing diagnostics and delivering personalized medical care. In the context of transportation, adapting to ever-changing roads and traffic conditions is critical for autonomous vehicles to make precise decisions, ultimately ensuring our safety on the road.
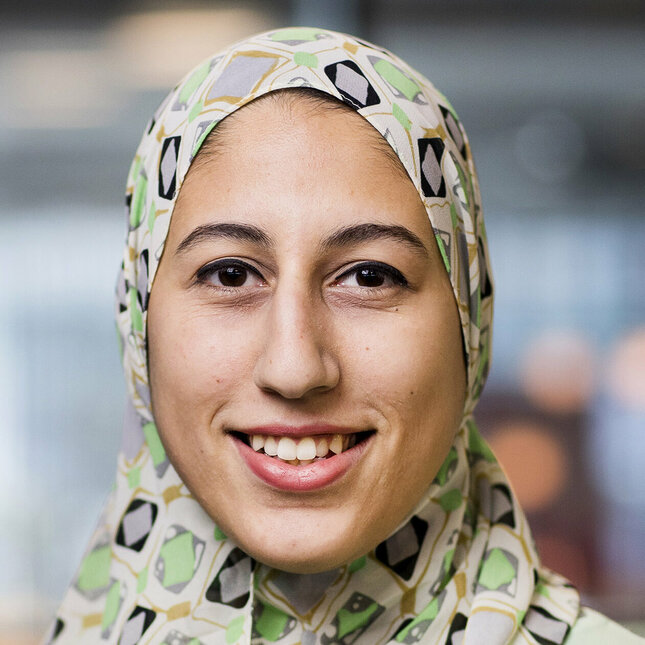
Environmental impact
This adaptability leads to the development of smarter, more accurate AI systems. However, as we strive for continual improvement in AI through such adaptability, we must also prioritize its environmental impact. The concept of Green AI emerges here, advocating for the creation of AI models that are environmentally conscious, aiming to reduce energy consumption and minimize resource usage while maintaining efficiency and accuracy.
The new methods developed by Sokar provide improvements across various aspects, including performance, memory efficiency, and computational efficiency. Leveraging these remarkable results, we can build new AI systems that potentially make an impact in every facet of our lives. Moreover, these advancements contribute to reducing energy consumption, steering us towards a sustainable future.
New continual learning paradigm
Sokar investigated the challenges that hinder current systems from continually learning and adapting, including catastrophic forgetting and loss of expressivity and plasticity. It showed that typical learning regimes do not directly fit this new continual learning paradigm. For instance, dense representations learned by typical learning lead to inference between old and new data and forgetting. Sokar’s research showed that sparse representations are more effective for this new regime. Moreover, optimizing the topology of the network on new data helps in learning more effective representations. Finally, it illustrated that changing data over time leads to unused network capacity. A periodic reinitialization of this capacity improves performance.
Title of PhD thesis: Learning Continually Under Changing Data Distributions
Supervisors: prof.dr. Mykola Pechenizkiy, dr. Decebal Mocanu.
Media contact
More on AI and Data Science
![[Translate to English:] All winners of the 2024 Research Awards. From left to right: Beatrice Federici, Francesca Grisoni, Marc Geers, Vincent Debets, Calum Ryan and Tugce Martagan. Photo: Bart van Overbeeke.](https://assets.w3.tue.nl/w/fileadmin/_processed_/5/1/csm_BvOF%202024_0613_DMH%20TUe%20Research%20Day%20-%20All%20Award%20winners_e4779eae60.jpg)
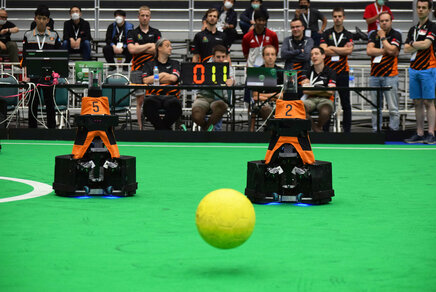
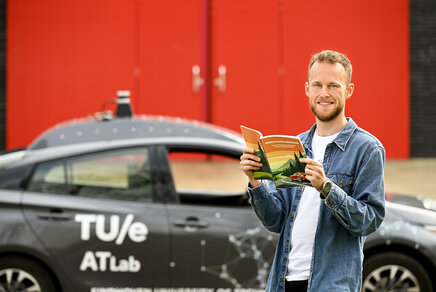
Latest news
![[Translate to English:] All winners of the 2024 Research Awards. From left to right: Beatrice Federici, Francesca Grisoni, Marc Geers, Vincent Debets, Calum Ryan and Tugce Martagan. Photo: Bart van Overbeeke.](https://assets.w3.tue.nl/w/fileadmin/_processed_/5/1/csm_BvOF%202024_0613_DMH%20TUe%20Research%20Day%20-%20All%20Award%20winners_e4779eae60.jpg)
![[Translate to English:] [Translate to English:]](https://assets.w3.tue.nl/w/fileadmin/_processed_/7/a/csm_image00007_be75479bcc.jpeg)
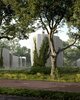
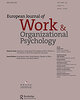
![[Translate to English:] [Translate to English:]](https://assets.w3.tue.nl/w/fileadmin/_processed_/b/7/csm_DSC02553_76391bbd00.jpg)
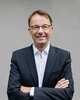