Algorithms for the monitoring of sleep disorders with wearables improved with deep learning
New algorithm developed to monitor sleep with wearables in patients with sleep disorders have been tested in the clinical setting
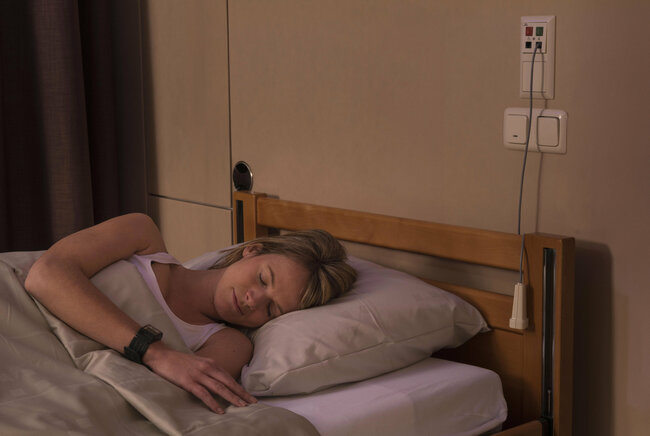
Approximately 10% of the world’s population are affected by insomnia, which is just one of a variety of serious sleep disorders. In a new study published in the journal Scientific Reports, TU/e researchers, along with colleagues from Sleep Medicine Center Kempenhaeghe, and Philips within e/MTIC, have developed a more reliable and better performing algorithm based on deep learning to better analyze sleep data for people with sleep disorders that has been measured using wrist-worn wearables.
According to the Irish proverb “A good laugh and a long sleep are the best cures in the doctor’s book.” While almost everyone enjoys a good joke once in a while, a good, long sleep is not something that everyone is capable of.
Given sleep’s importance, it is vital to monitor the sleeping patterns of patients whose sleep quality is disrupted by a sleeping disorder. In clinical settings, the gold standard is polysomnography (PSG), where an array of leads is connected to a patient. Algorithms developed to monitor sleep with wearables can serve as a strong complement to PSG, which due to its obtrusive nature is typically limited in use to one or at most two nights
“As clinicians, we would like to have greater insight into sleep patterns over longer periods of time, and that’s where wearable technologies and suitable algorithms can help,” says Sebastiaan Overeem, professor in the department of Electrical Engineering at TU/e and somnologist at Sleep Medicine Center Kempenhaeghe in Heeze.
A new algorithm
In the past, researchers and clinicians used actigraphy to gather some insight on longer term sleep/wake patterns for patients, which monitors body movements. But actigraphy has a key disadvantage. “Healthy persons move less when they sleep. However, persons suffering from insomnia can lie awake and be very still, a state that actigraphy deems to be sleep, when it is not,” notes Overeem.
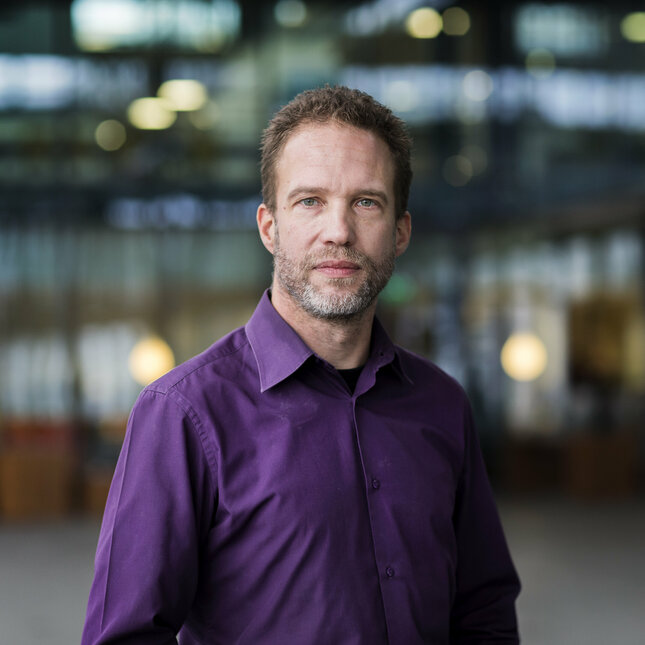
Researchers tried to solve this by measuring variations in heart rate using wrist-worn photoplethysmography (PPG), where light is used to measure changes in the volume of flowing blood.
“It’s one thing to have the technology, but it’s another thing to have the right tools to analyze the large amounts of data produced which can be used by a clinician to arrive at an accurate prognosis,” says Overeem. “In some of our previous work, we used machine learning algorithms to help with the analysis, but the approach required large amounts of computing power and time, as well as time-consuming manual marking of features in the sleep signals measured from a patient.”
In a new study recently published in the Nature journal Scientific Reports, Overeem and colleagues from TU/e, Sleep Medicine Center Kempenhaeghe, and Philips took another approach. Co-author Merel van Gilst, assistant professor from TU/e explains. “We started by ‘feeding’ a neural network with the raw movement and heart-beat data, along with gold standard PSG sleep data. Then, we let the algorithm automatically detect features of interest.”
This approach led to the training of a high-performing algorithm that required less computing power and time. “It’s now feasible to implement this algorithm in devices and cloud services for clinical use,” says van Gilst.
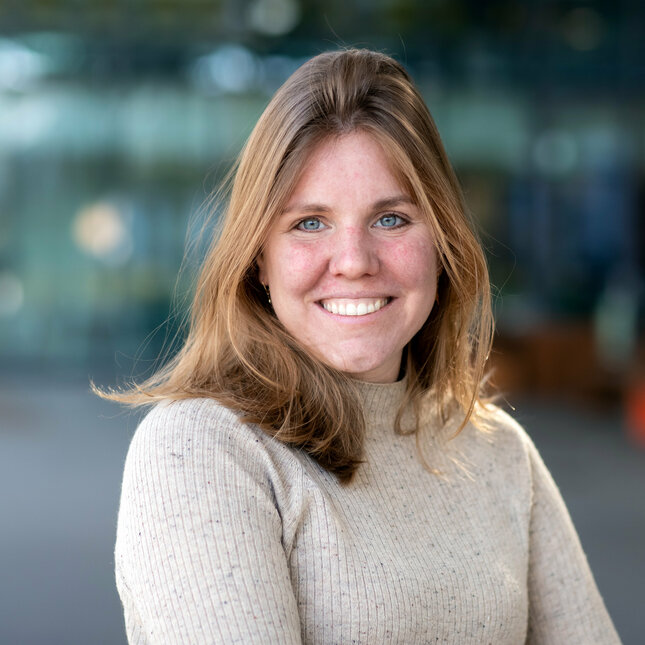
Consumer devices are not up to scratch
You be wondering why the researchers needed to create and train a new algorithm for sleep monitoring when there is a wide range of consumer devices out there that assess sleep quality using variations in heart rate. Just think of Apple devices and Garmin sports watches, for instance. Well, van Gilst highlights the main drawback with these devices for the clinical setting.
“The algorithms in consumer devices have been tested using small groups of healthy subjects who don’t have a sleeping disorder. In addition, these devices often assess sleep over large time windows to save battery life. As a result, fine details in the data are lost. Added to that, the performance and accuracy for different stages of sleep are quite low,” says van Gilst.
To develop trustworthy algorithms for sleep-monitoring devices in clinical settings, hundreds of sleep recordings measured with PSG in conjunction with data from wearable devices are required. This data should come from people of varying age and with all sorts of sleep disorders. Added to that, recordings need to be manually marked by sleep experts, and only then can the AI-based algorithms be created. “If you skip any of these steps, you will produce an inferior algorithm,” says Overeem. “And when it comes to monitoring sleep disorders, the effectiveness of the hardware is only as good as the algorithms designed to analyze the data they produce.”
Next steps
The new algorithms developed by the researchers could be used in wearable devices from different manufacturers, provided that the sensors in the devices are of high enough quality.
“Such technologies and algorithms could be very important additions to the toolkit of those assessing sleep disorders, which can strongly complement the gold standard approach that is PSG ,” notes van Gilst.
With the new algorithm in place, Overeem is excited by the future possibilities. “We can now start studies to really look at what these technologies bring to the clinical setting. For example, we are assessing long-term sleep patterns in patients with insomnia, in an attempt to better assess the severity and variability of the disorder and to further tailor treatment. .”
Added to that Overeem would like to use the data to measure data over a 24-hour period. “In this case, we could get a better idea about the negative effects of disrupted sleep on a patient in their day-to-day activities.”
Further information on the paper
Paper title: A computationally efficient algorithm for wearable sleep staging in clinical populations, Scientific Reports, (2023).
This project was performed within the Eindhoven MedTech Innovation Center (e/MTIC), and in a project involving Eindhoven University of Technology (TU/e), Kempenhaeghe, and Philips.
Media contact
More on Health
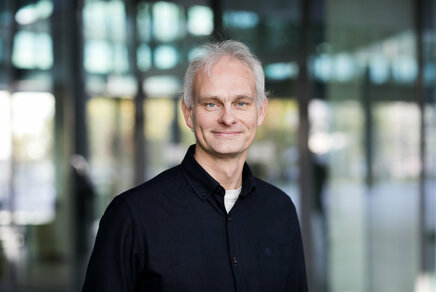
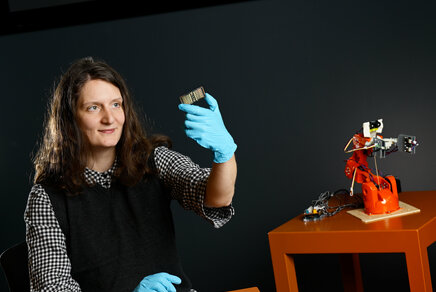
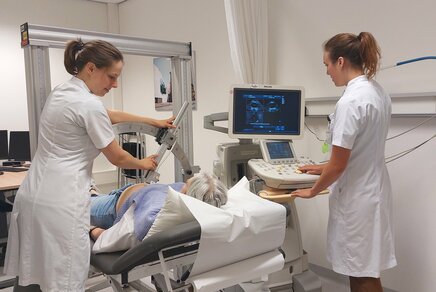
Latest news
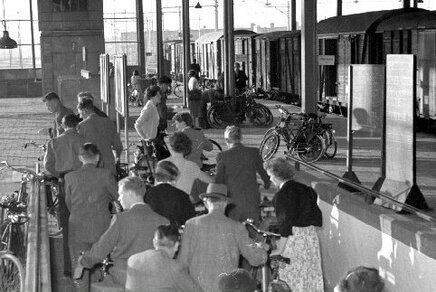
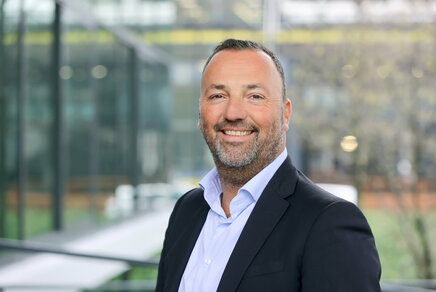
![[Translate to English:] [Translate to English:]](https://assets.w3.tue.nl/w/fileadmin/_processed_/e/0/csm_BvOF%202019_1031_BHF%20license%20TUe%20ILI%20copy_8a50884392.jpg)