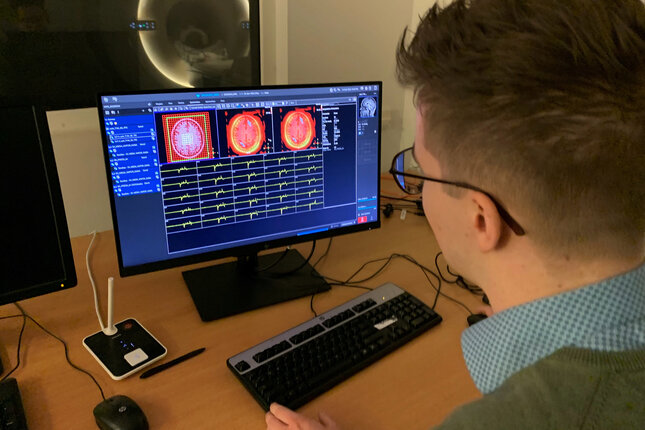
Spectralligence: Machine Learning for Spectroscopy Applications
By: Dennis van de Sande
Spectroscopy is a crucial technology in fields like chemistry, medicine, and environmental and safety services. It uses the electromagnetic spectrum to generate unique fingerprints of molecular structures. But, the process requires human experts to acquire, process, and analyse spectral data, which makes the overall workflow very complex for non-experts and relatively time-consuming. Spectralligence is changing that by introducing artificial intelligence (AI) into the spectroscopy process. Our team at TU/e is working with Philips, Maastricht UMC, and other spectroscopy companies to develop cross-domain validated neural networks that aims to revolutionize spectroscopy across industries. Our TU/e team focuses mainly on Magnetic Resonance Spectroscopy (MRS), a non-invasive technique used to study the metabolism of the human brain. With partners from different spectroscopic domains, we are able to bring fresh perspectives and innovative ideas to the table. Some of the AI solutions we're currently developing include generative models for spectral data generation, models for artifact removal, and classification tools for clustering or determining the quality of spectra. Visit our website to stay up-to-date with our latest achievements and join us on our journey to revolutionize spectroscopy with Spectralligence!
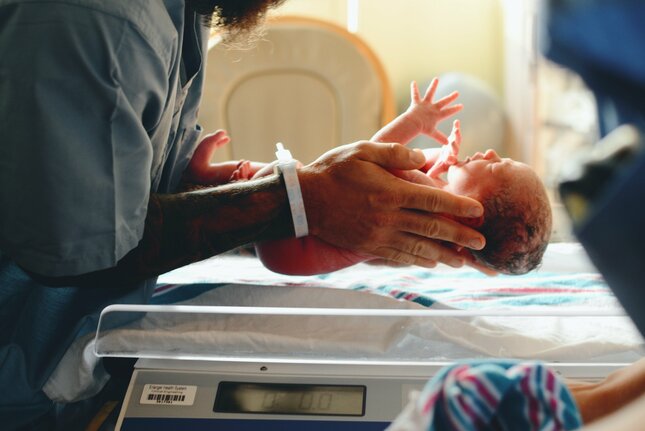
Early prediction and detection of perinatal complications
by: Ivar de Vries
Over two decades of collaboration between the Eindhoven University of Technology and the Máxima Medical Centre has resulted in a new method for monitoring unborn children during pregnancy and delivery. This method is based on electrodes and measures the fetal electrocardiogram and the uterine muscle activity (EHG). In this project, we aim to extend this monitoring method with the detection of fetal movement and the prediction of imminent preterm birth. Reduced fetal movement is considered an early predictor for complications, but currently no objective method for the quantification of fetal movement exists. By bridging this gap, we aim to give clinicians new tools for improving perinatal outcomes. Similarly, preterm birth is a worldwide leading cause of neonatal death, for which the outcome chances may be improved with the admission of lung maturation medicine. This admission is time-sensitive and overtreatment regularly occurs, which results in a clinical desire for methods capable of accurately prediction the time until spontaneous delivery. The EHG, however, is a highly complex signal and measurements are often
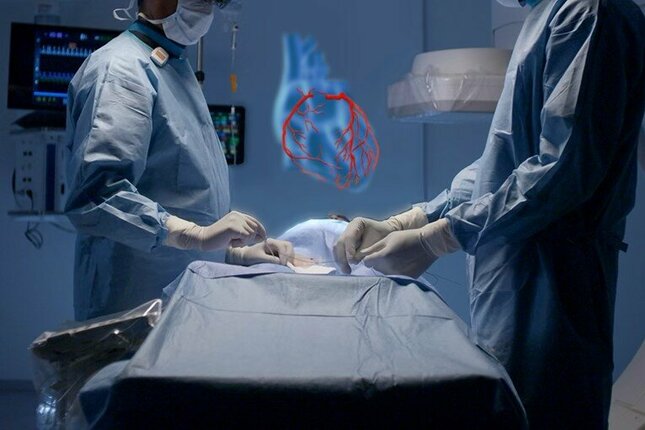
Artificial Intelligence in Percutaneous Coronary Interventions
By: Kirsten Maas and Amy Vermeer
Artificial Intelligence (AI) has the potential to benefit Percutaneous Coronary Interventions (PCI) procedures. In PCI, a stent is placed in the coronary artery to open up a local narrowing as result of plaque buildup. A successful PCI procedure requires an accurate characterization of the stenosis in terms of vessels dimensions and plaque morphology to select and deploy the appropriate stent for
the procedure. Inadequate stent selection or deployment may lead to recurrent symptoms and complications.
X-ray coronary angiography is the standard modality to assess the anatomy of coronary arteries and to guide PCI. Although mostly beneficial over other modalities, this 2D imaging technique suffers from the problem of foreshortening or a misperception of depth. As a result, stenoses cannot be accurately characterized in terms of size and shape. A 3D reconstruction of these 2D angiograms can overcome this problem. This project seeks to develop AI data-driven 3D reconstruction techniques for coronary X-ray angiography data. Challenges in the 3D reconstruction include automation, limited angular information and motion artifacts. The 3D reconstruction will be translated into meaningful clinical applications that can (automatically) quantify the lesion or support in stent selection or deployment.
The collaboration within the e/MTIC ecosystem allows us to develop and implement clinically-driven solutions driven by the Catharina hospital, while allowing us to exploit the technical knowledge and tools from our industrial partners at Philips.
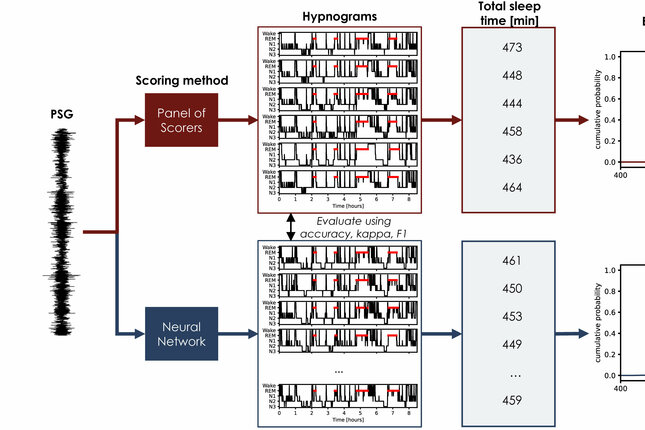
Deep Generative Learning for Uncertainty Estimation in Sleep Staging
By: Hans van Gorp
Gold-standard sleep staging as performed by human scorers comes with imperfect inter-rater agreement (~82.6%). Consequently, there is uncertainty about both the hypnogram and derived sleep statistics. These uncertainties can have significant consequences on both sleep diagnosis and basic sleep research. It would be desirable to model both uncertainties in automatic scoring. Deep learning algorithms have shown promise in this regard, but struggle to model the uncertainty about the overnight sleep statistics. To that end, we propose a novel technique based on conditional deep generative models, termed U-Flow. Rather than outputting a single hypnogram or hypnodensity per subject, U-Flow permits the sampling of multiple valid hypnograms, mimicking the process of a human panel. From this set of hypnograms, one can derive empirical distributions for each overnight statistic. This way, U-Flow is able to accurately model both the disagreement about the hypnograms and the overnight statistics, improving insights into the uncertainty of different sleep measures.
This research was performed within the e/MTIC collaboration framework, incorporating Eindhoven University of Technology, Philips Research, and Sleep Medicine Center, Kempenhaeghe Foundation.
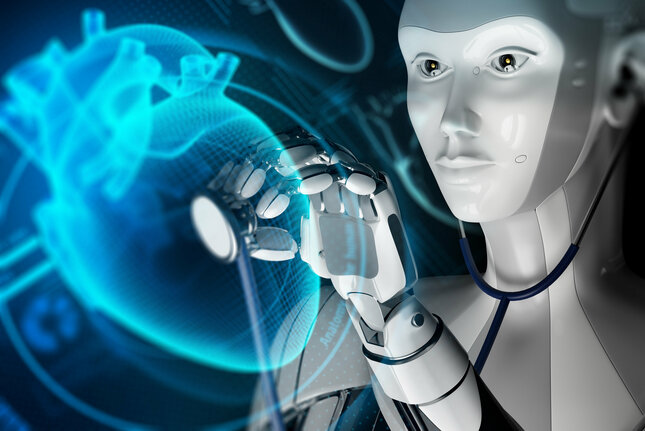
Advancing Cardiac Care through Interpretable AI (ACACIA)
By: Roy van Mierlo & Frederique de Raat
The field of critical care medicine has witnessed significant advancements in recent decades, particularly in the realm of hemodynamic monitoring. Advanced monitoring technologies, data analytics, and machine learning are at the forefront of transformative advancements in the intensive care unit. They have the potential to predict hemodynamic instability and redefine how and when we assess hemodynamic status.
In this research collaboration between the TU/e, the Catharina Hospital, and Philips we would like to go the extra mile. Besides early prediction of patient deterioration, streamlining of large data flows and hemodynamic intervention decision support are high-impact areas in the ICU to improve patient outcomes and clinical workflow. We aim to tailor hemodynamic intervention planning based on readily available high-fidelity data, and a deep understanding of the complex interaction between the heart and the arterial system.
By exploring the intersection of advanced technology, clinical medicine, and predictive analytics, this research seeks to illuminate new pathways for improving patient outcomes, fostering early